- On EdTech Newsletter
- Posts
- The Missing Discussion of AI Fine-Tuning by End Users
The Missing Discussion of AI Fine-Tuning by End Users
There's an emerging space between commercial chatbots using public data and complex AI model integrations

Was this forwarded to you by a friend? Sign up, and get your own copy of the news that matters sent to your inbox every week. Sign up for the On EdTech newsletter. Interested in additional analysis? Try with our 30-day free trial and Upgrade to the On EdTech+ newsletter.
For the past two years since OpenAI released ChatGPT 3.5, there has been a tremendous amount of attention placed on the consumer chatbot as the epitome of AI. And these tools have the intuitive, simplistic Google search aesthetic.

On the business, or enterprise, side, the Big Tech companies have added complex API access to their AI models. And we are already seeing larger organizations, perhaps most notably ASU, taking advantage of these interfaces that allow customization of data and prompts and outputs. But the access typically needs some real IT support to take advantage of the models, typically beyond your average educator’s skill set.
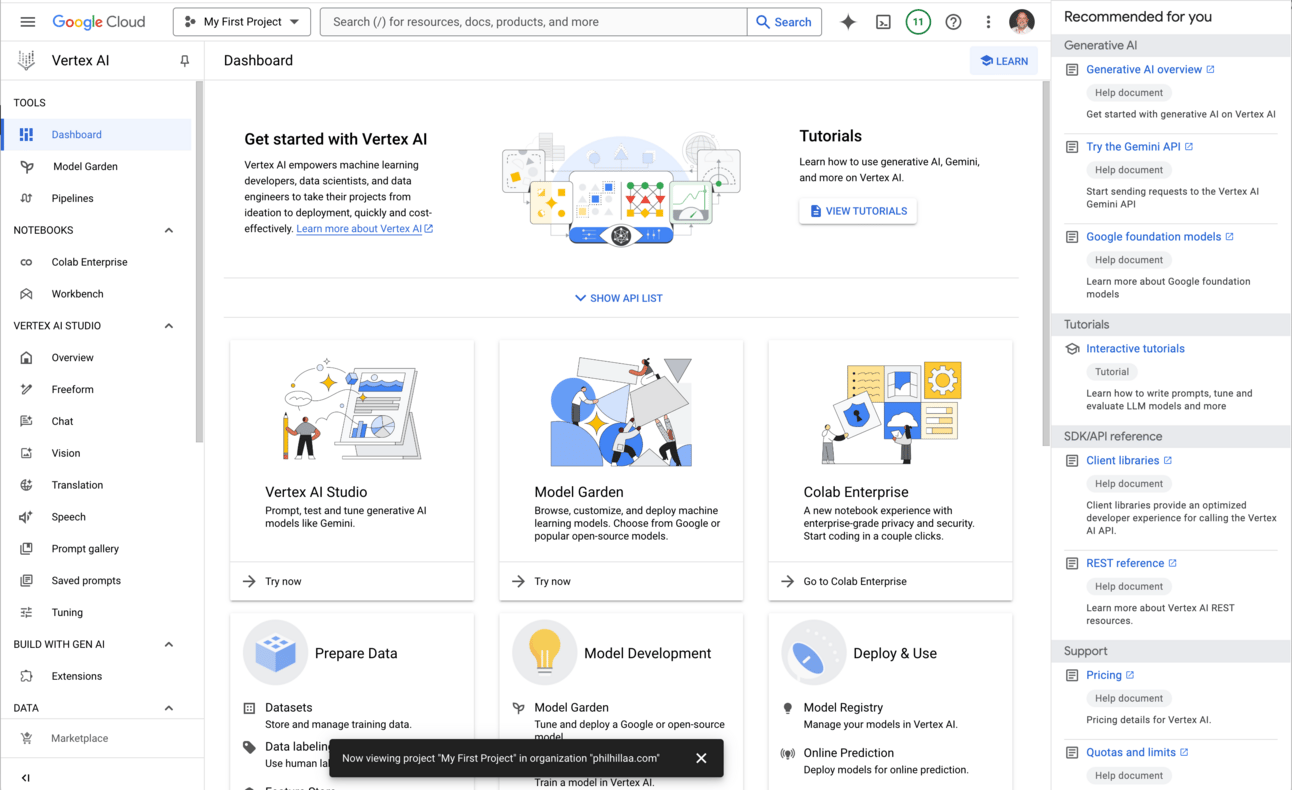
Despite generative AI being a development that won’t go away, it has been rejected by many in academic because neither end of the spectrum makes sense for them.
But there has been an emerging option over the past year that can allow consumer, or near end-user, access to customized AI knowledge based on fine-tuning. This development has not been discussed much, at least in EdTech circles, despite its ability to address some of the concerns over AI hallucinations and data privacy.
I am not arguing that fine-tuning resolves all problems, but I think it is worth exploring further.
The Basics
To keep us on the same page, generative AI using large language models (LLMs) first trains the model with massive computing resources and massive amounts of data. Most of that data comes from the open web, with a lot of data scraping, etc. This AI training leads to the large models - ChatGPT 3.5, ChatGPT 4o, Gemeni 1.5 Flash, Llama 3.2, etc. This training is typically a one-time event, with the knowledge base locked in to specific time frames (not based on current information).
At run time (the actual usage of the tools), typically a user enters a prompt in the associated chatbot, in a process known as AI inference. This is where the underlying AI uses the trained models - based on a passing of model weights - to come up with responses to the question or directions in the prompt.

Source: Mark Robins on LinkedIn: https://www.linkedin.com/pulse/difference-between-deep-learning-training-inference-mark-robins-mdq8c/
Four of the problems of generative AI usage include: